A Meta-Model of Enterprise AI Maturity: 4 Distinct Organizational Archetypes
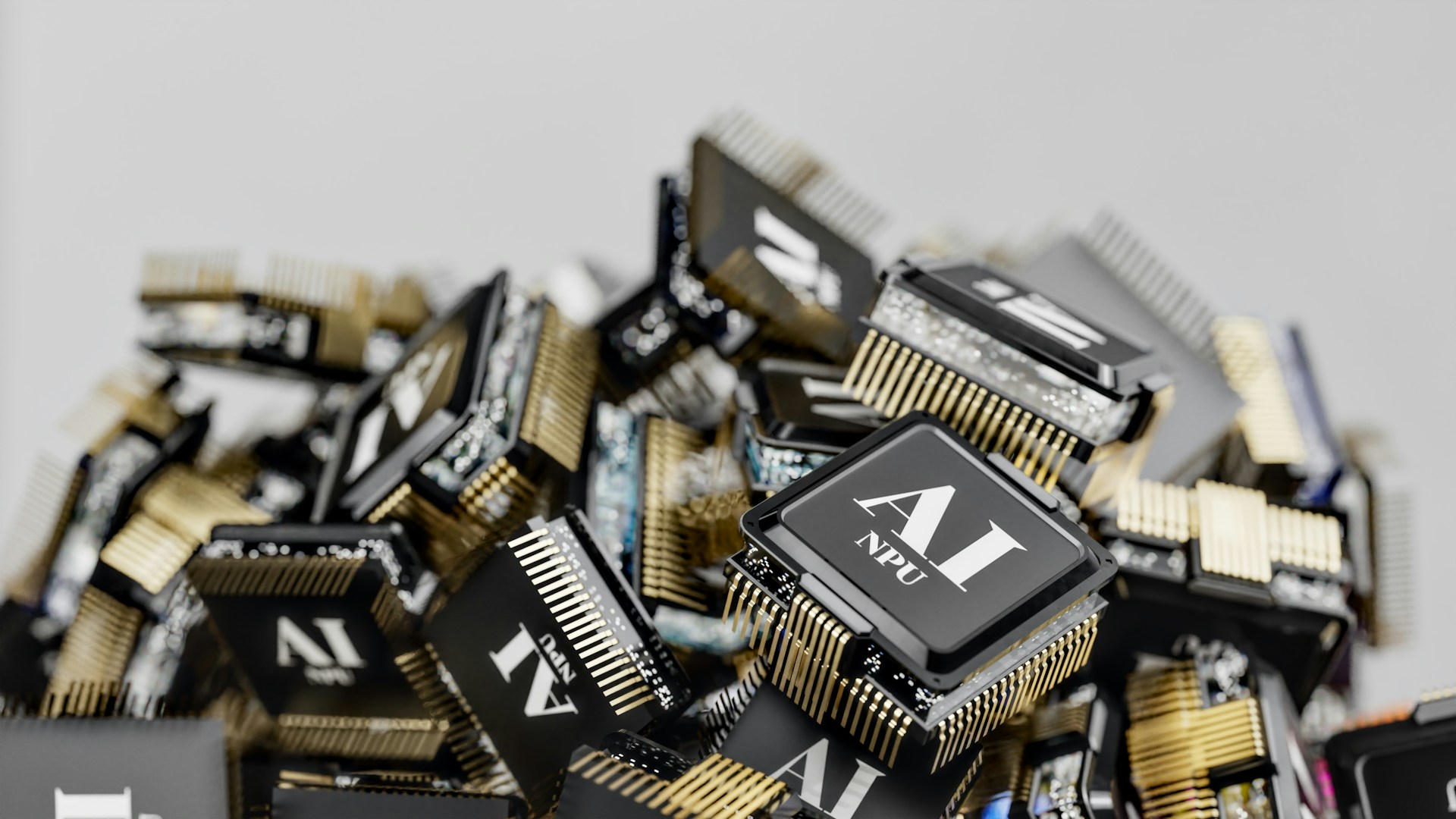
Is not just about technology; it is about culture, structure, and mindset.
Artificial Intelligence (AI) has shifted from being a mere asset to a driving force behind enterprise transformation, reshaping how businesses operate and compete in the digital age.
However, not all organizations adopt AI in the same way. Some cautiously experiment, while others fully integrate AI into decision-making and innovation. The key differentiator? AI is not just about technology; it is about culture, structure, and mindset.
This article introduces the Meta-Model of Enterprise AI Maturity, outlining four distinct organizational archetypes that define how enterprises adopt, scale, and evolve with AI. Inspired by MIT research on AI-driven business transformation, this model moves beyond technical implementation to highlight the systemic shifts in leadership, operations, and decision-making that truly define AI maturity.
Understanding where our organizations fit in this maturity spectrum is critical for navigating AI-driven growth. By assessing the AI archetype, we can pinpoint gaps, unlock new opportunities, and create a clear roadmap toward AI-powered innovation, resilience, and profitability.
Each archetype represents a distinct way in which an organization structures itself, manifests behavioral patterns, and interacts with AI. These are not just levels of AI maturity but deep-rooted systemic profiles that dictate how AI is adopted, resisted, or integrated.
1. Experiment and Prepare
(Centralized Control, Efficiency-Driven, Rule-Based AI Implementation)
This organization treats AI as a foundational experiment, embedding AI-driven automation and analytics to support early-stage decision-making and efficiency improvements. AI is used to test automation potential, build early AI capabilities, and establish basic AI governance frameworks.
Focus :
This stage is about building AI awareness, ensuring that leadership and employees understand AI’s potential and risks. Organizations work on defining acceptable-use policies, standardizing data, and setting up early AI governance structures.
The primary goal is to educate and prepare the workforce for more advanced AI integration.
Characteristics :
-
- Decision-Making: AI supports leadership with basic data insights, but decision-making remains primarily human-led.
- Behavioral Pattern: Employees are learning about AI, with limited hands-on interaction beyond early experiments.
- AI is used for data standardization and accessibility improvements.
- Initial acceptable-use policies and AI literacy initiatives are introduced.
- Focus on where human oversight is necessary in AI-driven processes.
AI Culture Readiness behaviors :
- Employees are aware of AI but lack deep engagement.
- Leadership is hesitant to fully trust AI for business decisions.
- AI is used for basic data-driven decision support, but not yet embedded in workflows.
Organizational Challenges :
- Slow AI adoption due to workforce skepticism.
- Lack of clear AI business cases, making it difficult to justify further investments.
- Data fragmentation, limiting AI’s effectiveness.
Percentage of Firms at This Stage :
🔹 28% of organizations. A significant portion of companies are in this early stage, indicating a widespread need for AI literacy, experimentation, and foundational policy-making.
Financial Impact :
Growth: -12.6 pp below industry average. AI adoption at this stage is not yet translating into financial growth, as organizations are still experimenting with use cases.
Profit: -9.6 pp below industry average. Investment in AI infrastructure and training has not yet yielded tangible financial benefits
2. Build Pilots and Capabilities
(Specialist-Led, Knowledge-Based, AI as an Assistant for High-Skilled Professionals)
This organization pilots AI use cases and starts integrating AI into specific business functions. AI is used to augment specialists, improve automation, and provide deeper analytics for decision-makers.
Focus :
The primary focus is testing AI’s real-world application. Organizations move beyond education and begin identifying high-impact areas where AI can improve business functions. They run pilot projects and measure AI’s effectiveness in improving efficiency and decision-making.
Characteristics :
- Decision-Making: AI advises experts and managers, but final decisions remain human-driven.
- Behavioral Pattern: Employees engage with AI in select functions, mostly as decision-support tools.
- AI is used to simplify and automate specific processes.
- Early data-sharing initiatives and API integration emerge.
- Adoption of out-of-the-box AI models for workplace augmentation.
AI Culture Readiness behaviors :
- Employees begin trusting AI in specialized roles but still hesitate in broader applications.
- Leadership is willing to fund AI pilots but demands clear ROI.
- AI is viewed as a technical enhancement rather than an enterprise-wide necessity.
Organizational Challenges :
- AI remains siloed—limited to specialists and not accessible across teams.
- Difficulties scaling successful pilots due to infrastructure limitations.
- Resistance from employees fearing AI automation risks.
Pourcentage d’Entreprises à ce Stade :
🔹 34% of organizations. This stage is the most common, reflecting that many organizations are still experimenting and refining AI adoption strategies..
Financial Impact :
Growth: -3.5 pp below industry average. While AI pilots show potential, they have not yet reached enterprise-wide impact.
Profit: -2.2 pp below industry average. Some financial returns are seen, but scaling challenges prevent significant profit increases.
3. Develop AI Ways of Working
(Decentralized, Dynamic, AI as a Real-Time Learning and Feedback System))
This organization fully integrates AI into core business operations, embedding AI into scalable platforms and workflows. AI assists in real-time decision-making, automation, and organizational learning.
Focus :
The focus shifts from testing AI to scaling AI across business processes. Organizations invest in platforms, automation, and AI dashboards to create a more data-driven and adaptive work culture.
Characteristics :
- Decision-Making: AI plays an active role in business decisions, offering predictive insights, process automation, and continuous feedback.
- Behavioral Pattern: Employees rely on AI-assisted workflows, where automation enhances productivity but still allows for human oversight.
- AI-driven systems improve process efficiency, reducing redundancy and optimizing resource allocation.
- Adoption of real-time AI analytics dashboards to monitor performance, trends, and bottlenecks.
- Integration of AI-powered workflow automation across departments to streamline operations.
AI Culture Readiness behaviors :
- Employees are actively using AI in their daily work, with increasing trust in AI-generated insights.
- Leadership relies on AI-driven analytics for strategic and operational decisions.
- The organization invests in AI training to ensure continued workforce adaptability.
Organizational Challenges :
- Balancing automation and human oversight, ensuring AI enhances rather than replaces human roles.
- Infrastructure complexity, requiring significant investment in AI governance, platforms, and cybersecurity.
- Maintaining AI accuracy and reliability, ensuring AI models remain relevant and unbiased over time.
Percentage of Firms at This Stage :
🔹 31% of organizations. A growing number of enterprises recognize AI’s business potential and work to integrate it at scale.
Financial Impact :
Growth: +11.3 pp above industry average. AI begins to drive efficiency and revenue growth.
Profit: +8.7 pp above industry average. Investments in AI infrastructure and process automation start yielding tangible financial returns.
4. AI Future Ready
(Fully AI-Symbiotic, AI and Humans as Co-Evolutionary Partners in Innovation and Growth)
This organization leverages AI as a transformative force, embedding AI into strategic leadership, business model innovation, and product development. AI is used not just for optimization but to create new revenue streams, automate strategic decisions, and enhance human creativity.
Focus :
AI is no longer an enhancement—it becomes a core component of business growth and competitive strategy. Organizations at this stage develop proprietary AI models, sell AI-driven services, and use AI for continuous innovation.
Characteristics :
- Decision-Making: AI is deeply integrated into strategic planning, providing autonomous recommendations and assisting leaders in shaping business direction.
- Behavioral Pattern: Employees collaborate with AI as co-workers, using AI-driven insights to augment human creativity, innovation, and high-level decision-making.
- AI is applied to business model reinvention, enabling entirely new revenue streams based on AI-powered services or platforms.
- Continuous AI experimentation and feedback loops, ensuring ongoing refinement and enhancement of AI-driven initiatives.
- AI-driven personalization and hyper-automation create seamless, intelligent customer experiences.
AI Culture Readiness behaviors :
- Employees are highly AI-literate, using AI to enhance problem-solving, creativity, and decision-making.
- Leadership treats AI as a co-pilot for innovation, actively integrating AI into company-wide strategic initiatives.
- The company is data-centric, ensuring all operations and business decisions are rooted in AI insights.
Organizational Challenges :
- Ethical and regulatory risks, requiring strong AI governance and compliance measures.
- High infrastructure and R&D costs, making AI development and deployment an ongoing investment.
- Workforce evolution, requiring continuous reskilling and alignment between human roles and AI-driven automation.
Percentage of Firms at This Stage :
🔹 7% of organizations. Few companies have fully integrated AI at this level, but those that do see exponential benefits.
Financial Impact :
Growth: +17.1 pp above industry average. AI drives new revenue streams and business model transformations.
Profit: +10.4 pp above industry average. AI-fueled efficiency and innovation create sustained profitability.
Conclusion
The four organizational archetypes provide a blueprint for businesses to assess where they stand and define the next steps for their AI transformation. Whether your organization is just experimenting or already leveraging AI at scale, the key to sustained AI maturity lies in continuous adaptation, workforce alignment, and a systemic approach to AI integration.
References:
- MIT CISR 2022 Future Ready Survey (N=721) and interviews in 2024 with sixteen executives at nine enterprises.
- https://mitsloan.mit.edu/ideas-made-to-matter/whats-your-companys-ai-maturity-level?utm_source=instagram&utm_medium=social&utm_campaign=aimaturity
__
Viorel Bucur is the co-founder of Upscale Paris and The Scale Project podcast.
Entrepreneur and Team Coach (ICF) with over a decade of experience in behavioral sciences, organizational systems, and tech entrepreneurship, he helps leaders and organizations navigate digital transformation, AI adoption, and organizational change.
Passionate about human potential and leadership development, Viorel is dedicated to shaping the next generation of conscious and high-impact leaders, guiding them through transformational journeys that redefine the way they work, learn, and lead.